This article considers the estimation of damped linear oscillators with psychological data. The formulas of the new method presented in this chapter, which related the τ-conditional and τ-corrected estimates of model parameters, are described.
Abstract
How do individuals change over time? When? Why? These loaded questions are becoming more common to a range of psycho logical contexts and ones that address fundamental psychological issues (Nesselroade, 1991; Nesselroade & Ram, 2004). Examination of individuals through intensive, longitudinal measurement is one way in which researchers will begin to address questions regarding individual change over time. Historically the examination of change over time has been successfully addressed through techniques such as differential equation models and concepts such as those of dynamical systems.
Application of differential equation modeling and dynamical systems to psychological science has proved to be difficult. The difficulty can be attributed to at least two sources. The first is due to the fact that the under lying processes in psychology are likely to be more intricate than those for which these methodologies were developed, that is, the physical and natural sciences (Boker, 2002). This problem will only be remedied as research in psychology becomes more adept at describing change over time. The second source of difficulty are the data common to psychology, which are not measured with the precision and accuracy available in classical physics (Boker, 2002). This creates a host of problems, one of which is the presence series. The formulas of the new method presented in this chapter, which related the τ-conditional and τ-corrected estimates of model parameters, are then described. Finally, the results from simulation work are presented, including time series with and without oscillating components.
Code and Other
ContentOscillatorEstimation.R
Citation
Deboeck, P. R., Boker, S. M. (2009). Unbiased, Smoothing–Independent Estimation of Oscillators in Psychology. In S. Chow, E. Ferrer & F. Hsieh (Eds.), Statistical Methods for Modeling Human Dynamics: An Interdisciplinary Dialogue, pp. 179–212. New York, NY: Taylor & Francis Group.
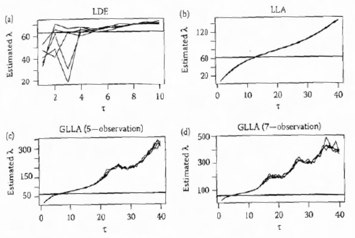