Using embedded and observed data matrices, a statistical approach to differential equation modeling is presented. This approach appears robust to short, poorly sampled time series with large proportions of measurement and dynamic error, as is common in psychological research.
Abstract
Complex intraindividual variability observed in psychology may be well described using differential equations. It is difficult, however, to apply differential equation models in psychological contexts, as time series are frequently short, poorly sampled, and have large proportions of measurement and dynamic error. Furthermore, current methods for differential equation modeling usually consider data that are atypical of many psychological applications. Using embedded and observed data matrices, a statistical approach to differential equation modeling is presented. This approach appears robust to many characteristics common to psychological time series.
Code and Other Content
Observed&Expected.R
Citation
Deboeck, P. R. & Boker, S. M. (2010). Modeling Noisy Data with Differential Equations using Observed and Expected Matrices. Psychometrika, 75 (3), 420–437.
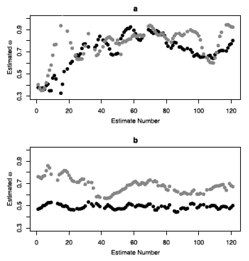